by Dr Xiaodan Xing
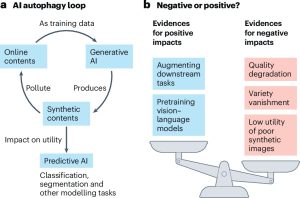
The latest paper by the Yang Lab, published in Nature Machine Intelligence, delves into a pressing issue in AI development: what happens when generative models train on their own synthetic data? This phenomenon, known as AI autophagy, leads to model collapse, loss of diversity, and ethical risks.
As the awareness of AI autophagy grows, so does the call for a comprehensive framework to understand, detect, and mitigate its effects. Our research brings together conflicting findings, theoretical perspectives, and empirical evidence to highlight the risks and propose potential solutions.
One key takeaway is that technical fixes alone, like watermarking or detection methods, aren’t enough. To truly address AI autophagy, we need a holistic approach that integrates technical solutions with robust regulatory oversight. Without proper policies and governance, even the most advanced technical solutions could fail to scale effectively.