We welcome back guest blogger, Manya Bhargava, to explain this year’s Chemistry Nobel Prize, awarded to David Baker, Demis Hassabis and John Jumper, for the development of AlphaFold! Developed by Google DeepMind and EMBL-EBI, AlphaFold is an AI system that predicts protein structures.
Imagine you’ve just bought a new Lego set. You’re super excited to get started. You have the pieces arranged, and all you need are the instructions to tell you how to fit them together. But someone seems to have played an awful prank on you. Instead of instructions in the box, you find a long list of clues about how the blocks need to fit together. Blue blocks can only connect to yellow ones, flat bricks can only be on the top… and so on. You know what the final model should look like, but this sure makes it much harder to build!
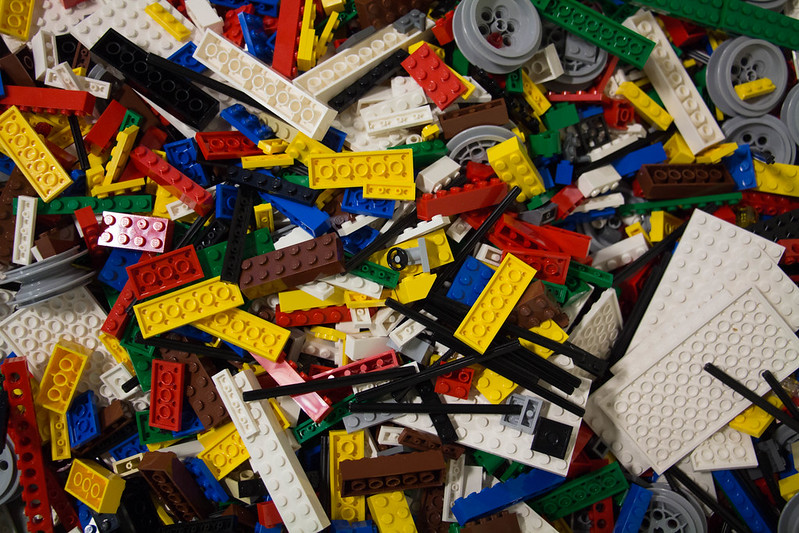
Much like Lego, proteins are made up of building blocks which all fit together in a very specific way to form the right structure. Scientists also have a list of clues as to how the blocks fit together. These are the physical forces underpinning how they interact with each other. For decades we’ve been trying to figure out the ‘instructions’, so that we can take the building blocks of any protein and predict what the resulting structure would be. We call this the Protein Folding Problem.
The protein folding problem (PFP)
Proteins play a critical role in our bodies. They form antibodies to protect against disease and enzymes which speed up vital biological reactions. The function of a certain protein depends on its unique composition and shape.
Smaller ‘blocks’, called amino acids, link together by peptide bonds forming a polypeptide chain. This chain folds into a specific shape, called the native structure, which depends on the properties of the amino acids. There are 20 different types of amino acids, and each chain will have a different combination of these to form a unique protein.
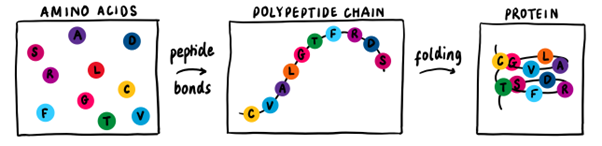
The ability to predict the shape of a folded protein from its amino acid sequence is an incredibly powerful tool. Applications include designing new drugs to target protein-related diseases like Alzheimer’s and Parkinson’s. This is, however, a very difficult task for classical computers. The nature of each amino acid, along with their interactions with each other and their environment, must be considered to find the protein’s native structure, which leads to immensely complex calculations.
AlphaFold
Recently, researchers turned to machine learning to solve the PFP by training AI to predict structures using a huge database of known protein shapes. This led to the release of AlphaFold in 2021 (amongst similar technologies) which finds patterns between similar protein sequences. AlphaFold’s success was groundbreaking. Making possible to map the structure of 98.5% of proteins in the human body when given the amino acid sequence.
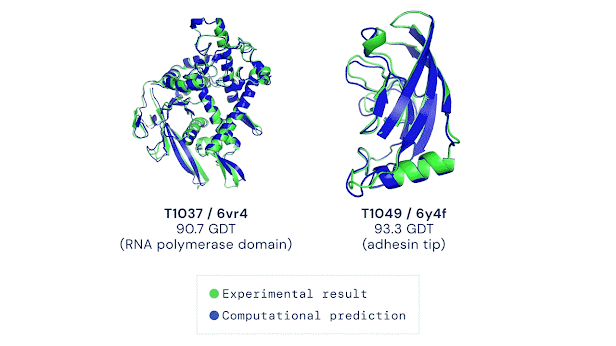
However, there are still challenges in the art of protein folding prediction. AlphaFold is trained on a database of known proteins. Some of its success depends on the knowledge of these training structures, and not an understanding of the underlying physics behind folding. It’s like memorising loads of Lego models and copying parts of their structures which use the same bricks that you have, rather than using the list of rules you’ve been given.
This method could lead to issues in predicting dynamic proteins which change shape to perform their functions, dealing with mutated (often disease-causing) proteins, and inaccuracies in those which don’t have close relatives in the database – like proteins which may be designed for new drugs. Although AlphaFold was a revolutionary step forward, there is space for other techniques to fill in the gaps.