For most physical illnesses, there are objective tests to determine what a patient’s issue is. Currently, diagnosis of mental health conditions is more subjective, as it relies on patient’s descriptions of their own symptoms. What if digital tools could identify biomarkers which were clearly linked to specific mental illnesses?
By Naveesha Karunanayaka
The aim of new diagnostic tools is to gain accurate, objective information regarding a patient’s symptoms and general well-being. With current technology and advances, new methods provide hope for more accurate diagnosis and treatment.
A new era for diagnosis
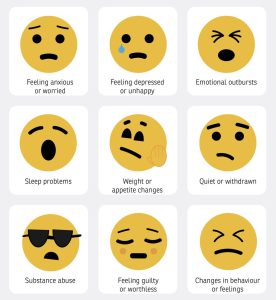
Smartphones, smart watches and apps enable us to assess mood, cognition and behaviour through the data collected every day by our personal devices. This is known as digital phenotyping. Changes in activity, sleep or device use can indicate a change to mental wellbeing. When the device detects a pattern that it believes is due to a certain mental illness, it can send a signal to the doctor for action. This has the potential to detect symptoms of an illness early on, so that treatment can be administered as soon as possible. Conditions could be controlled more effectively and negative impacts reduced.
However, there are challenges facing this technology, despite the actual devices’ good usability. For example, there needs to be a clear actionable reading that allows a signal to be sent to the doctor. For daily activity and sleep this can be achieved fairly easily, as changes are clear. But changes to keystroke and scrolling patterns are less distinct.
Artificial Intelligence and Machine Learning in diagnostic tools
Artificial Intelligence (AI) and Machine Learning (ML) techniques are increasingly used to analyse facial expressions and in voice recognition. Researchers are now able to design ML algorithms that can distinguish patients with mental health conditions. The challenge is to distinguish between different conditions. Despite this, a team at Tokyo University claims to be able to differentiate between multiple psychiatric disorders, including autism spectrum disorder and schizophrenia. They built an ML algorithm to find patterns in a data set of 200 MRI brain scans from patients with established diagnoses, then used this algorithm to predict the diagnosis for a new group of 43 patients with up to 85% reliability.
Broadening this method to a wider group is challenging because MRI scans differ between machines, equivalent to capturing the same photo on two different phone brands, resulting in a slightly different outcome. This is obviously a limitation that needs to be overcome before the technique can be put into use.
Translating brainwaves
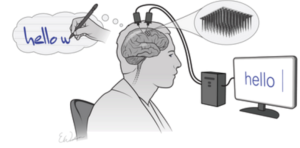
It is now possible for a computer to “read” brain activity. This technique is known as brain-computer interfaces (BCI). This translates your brain activity by converting the electrical impulses into signals that a computer can understand. Currently a BCI can only pick up simple brain messages. This includes emotional states, or which movements you intend on making, or whether you are going to say, ‘yes’ or ‘no’. However, now, researchers and companies are exploring whether they can decode these electrical signals to give an insight into a person’s thoughts or use brain activity to control a remote device. But this does raise ethical issues and there is concern about the potential effects of BCI on personality and autonomy.
Intrusive devices
Using a physical tool to help diagnose a condition in the brain is extremely difficult. Any tools used to monitor brain chemicals and neurotransmitters need to be safe, have high spatiotemporal resolution, and have high selectivity and sensitivity. Methods also need to be fast and minimally invasive. Another challenge is the nature of the blood-brain barrier, the membrane around the brain which prevents blood coming into contact with neurons. This means that chemical analyses of blood samples do not accurately reflect brain composition; it is also difficult to deliver drugs to the brain non-invasively. This is an active area of research at Imperial, so hopefully there will be developments in the near future.
Conclusions
Technological solutions to aid diagnosis of mental illnesses is a rapidly developing area. However, these tools are not yet refined, and need more research and evidence from studies on a large scale before they can be put to use. Some techniques are less invasive than others, and it would be expected that these will be more prominent in the future as we aim for less intrusive medical procedures. However, progress in any one of the possibilities above would be welcomed by many and could make a real difference to many lives.
This is part of a blog series on the molecular science of mental health. Read more about anxiety and depression, diagnostic tools and digital twins.
Further reading
Health Direct (n.d.). Nine signs of mental health issues. Available from: https://www.healthdirect.gov.au/signs-mental-health-issue [Accessed 9th August 2021].
Hirschtritt, M. E., & Insel, T. R. (2018). Digital Technologies in Psychiatry: Present and Future. Focus (American Psychiatric Publishing). 16(3): 251–258. doi: https://doi.org/10.1176/appi.focus.20180001
Matthews, K. (2019). 6 Innovations That Have Drastically Changed Mental Healthcare. Available from: https://www.hcinnovationgroup.com/population-health-management/telehealth/article/21118902/6-innovations-that-have-drastically-changed-mental-healthcare [Accessed 8th August 2021].
The Scientist. (2021). Brain-Computer Interface User Types 90 Characters Per Minute with Mind. Available from: https://www.the-scientist.com/news-opinion/brain-computer-interface-user-types-90-characters-per-minute-with-mind-68762 [Accessed 9th August 2021].
University of Tokyo (2020). Future mental health care may include diagnosis via brain scan and computer algorithm. Available from: https://www.sciencedaily.com/releases/2020/08/200817104247.htm [Accessed 9th August 2021].
Widge, A. S., Dougherty, D. D., and Moritz, C. T. (2014). Affective Brain-Computer Interfaces As Enabling Technology for Responsive Psychiatric Stimulation. Brain computer interfaces (Abingdon, England). 1(2): 126–136. doi: https://doi.org/10.1080/2326263X.2014.912885