As part of #LoveData24, the Research Data Management team had a chance to catch up with Yves-Alexandre, Associate Professor of Applied Mathematics and Computer Science at Imperial College London, who also heads the Computational Privacy Group (CPG). The CPG are a young research group at Imperial College London studying the privacy risks arising from large scale behavioural datasets. In this short interview we discussed the interests of the group, the challenges of managing sensitive research data and whether we need to reevaluate what we think we know about anonymisation.
How did you become involved with the Computational Privacy Group (CPG)?
Yves-Alexandre de Montjoye (YD)
So, my career as a researcher started when I was actually doing my master’s thesis at the Santa Fe Institute in New Mexico. That was in in 2009. This was pre-A.I. and the beginning of the Big Data era. People were extremely excited about the potential for working with large amounts of data to revolutionise the sciences, ranging from social science to psychology, to urban analytics or urban studies and medicine.
So many things suddenly became possible and people were like, ”this is the microscope”, or any other kind of analogy you can think of in terms of this being a true revolution for the scientific process. Some even went as far as saying, “this is the end of theory, right?” or “this spells the end of hypothesis testing. The data are going to basically speak for themselves”. There was a huge hype of expectation which, as time went on gradually decreased and eventually plateaued to what it is now. It did have a transformative impact on the sciences but to me it became quite obvious working with these data as a student, just how reidentifiable all these types of data potentially were.
Back in the days, we were looking at location data across the country and on the one hand, everyone was talking about how the data were anonymous. As a student, I was working with the data and I could see people moving around on the map, so to speak. And it just blew my mind. It didn’t seem like it would take very much for these data to not be anonymous anymore.
Anonymisation and the way we’ve been using it to protect data have been well documented in the literature. There has also been extensive research on how to properly anonymise data. I think what has taken a bit of time for people to grasp is that anonymisation, in the context of big data, is its own new, different question, and that actually a lot of the techniques that had developed from around 1990 to 2010 were basically not applicable to the world of big data anymore.
This is mostly due to two factors. The first one is just the sheer amount of data that is being collected about every single person in any given dataset that we are interested in, from social science to medicine.
Combining these with social media and the availability of auxiliary data (meaning data from an external source, such as census data) means that not only are there a lot of data about you in those datasets, but there are also a lot of data about you that can be cross-referenced with sources elsewhere to reidentify you. And I think what took us quite a bit of time to get across to people was that this was a novel and unique issue that had to be addressed. It’s really about big data and the availability of auxiliary data. I think that’s really what led a lot of our research into privacy. Regarding anonymisation, we are interested in the conversation around whether there is still a way to make it work as intended given everything we know or do we need to invent something fundamentally new. If that is the case, what should our contribution to a new method look like?
At the end of the day, I think the main message that we have is that anonymisation is a powerful guarantee because it is basically a type of promise that is made to you that the data are going be used as part of statistical models, et cetera, but they’re never going to be linked back to you.
The challenge lies in the way we go about achieving this in practice. Deidentification techniques and principles such as K-anonymity are (unfortunately) often considered a good way of protecting privacy. These techniques which, basically take a given dataset and modify it in one way or another, might have been considered robust enough when they were invented in the 90s and 2000s, but because of the world we live in today and the amount of data available about every single person in those datasets, they basically fall short.
There is a need for a real paradigm shift in terms of what we are using and there are a lot of good techniques out there. Fundamentally, the question comes down to what is necessary for you to make sure that the promise of anonymisation holds true, now and in the future.
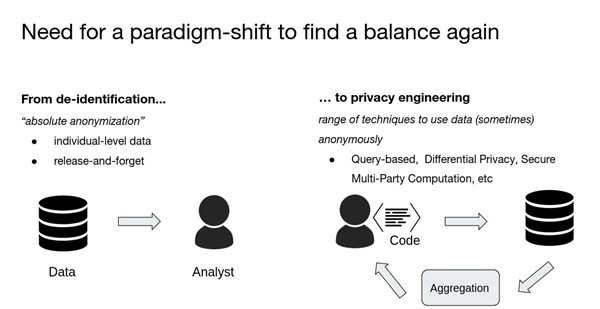
Could you talk to me a little bit about the Observatory of Anonymity and what this project set out to achieve? And then as a second part of that question, are there any new projects that you’re currently working on?
YD: The Observatory of Anonymity comes from a research project published by a former postdoc of mine, and the idea is basically to demonstrate with very specific examples to people, how little it takes to potentially reidentify someone.
Fundamentally, you could spend time trying to write down the math to make sense of why you know for a certain number of reasons that a handful of pieces of information are going to be sufficient in linking back to you. The other option is to look at an actual model of the population of the UK. As a starting point, we know that there are roughly 66 million people living across the country. Even if you take London, there are still 10 million of us. And yet, as you start to focus on a handful of characteristics, you begin to realise very quickly, that those characteristics, when put together, are going to make you stand out and a significant fraction of the time that can mean that you will be the only person in all of the country to match those sets of characteristics.
The interesting part is what do we do?
You’re working within Research Data Management and your team are increasingly dealing with sensitive data and the question of how they can be safely shared?
Clearly there are huge benefits to data being shared in science, in terms of verifying research findings and reproducing the results and so on. The question is how do we go about this? What meaningful measures can you put in place to ensure that you sufficiently lower the risks of harmful disclosure in such a way that you know that the benefit of showing these data will clearly outweigh those risks?
I think from our perspective, it’s really about focusing on supporting modern privacy-ensuring approaches that are fit for purpose. We know that there are a range of techniques; from controlled access to query-based systems, to some of the encryption techniques that, depending on the use case, who needs to access your data, and the size of your dataset would allow someone to use your data, run analysis, and replicate your results fully without endangering people’s privacy. For us, it’s about recognising the right combination of those approaches and how we develop some of these tools and test them.
I think there has been a big push towards open data, under the de-identification model, for very good reasons. But this should continue to be informed by considerations around appropriate modern tools, to safeguard data while preserving some utility. Legally at least, you cannot not care about privacy and if you want to care about privacy properly, this will affect the utility. So we need to continue to handle questions around data sharing on a case-by-case basis rather than imply that everything should be fully open all of the time. Otherwise this will be damaging to the sciences and to privacy.
Yes, it is important to acknowledge that that tension between privacy and utility of research data exists and that a careful balance needs to be struck but this may not always be possible to achieve. This is something that we try to communicate in our training and advocacy work within Research Data Management services.
We have adopted a message that can hopefully be helpful (and which originated from Horizon Europe[1]), which states that open science operates on the principle of being ‘as open as possible, as closed as necessary’. In practice this means that results and data may be kept closed if making them open access is against the researcher’s legitimate interests or obligations to personal data protection. This is where a mechanism such as controlled access could play a role.
YD: Just so. I think you guys have quite a unique role to play. A controlled access mechanism that allows a researcher to run some code on someone else’s data without seeing the data on the other hand requires systems of management, authorisation and verification of users, et cetera. This is simply out of the reach of many individual researchers. As a facility or as a form of infrastructure however, this is actually something that isn’t too difficult to provide.
I think France has something called the CASD, which is the Center for Secure Access to Data (or Centre d’Accès Sécurisé aux Données) and this is how the National Institute of Statistics and Economic Studies (INSEE) is able to share a lot of sensitive data. Oxford’s OpenSAFELY in the UK is another great example of this. They are ahead in this regard. We need similar mechanisms when it comes to research data to facilitate replicability, reuse and for validating and verifying results. It is absolutely necessary. But we need proper tools to do this and it’s something that we need to tackle as a collective. No individual researcher can do this alone.
What in your experience are common misconceptions around anonymisation in the context of research data?
YD: I think the most common misunderstanding is a general underestimation of the scale of data already available. Concerns often revolve around a notion of, could someone search another person’s social media and deduce a piece of information to reidentify them in my medical dataset? In the world of big data, I would argue that what we strive to protect against also includes far stronger threat models than this.
We had examples in the US in which you had right wing organisations with significant resources buying access to location data, matching them manually, potentially at scale with the travel record, and other pieces of information they could find about clerics to potentially identify them in this dataset, in an attempt to see if anyone was attending a particular seminar[2].
We had the same with Trump’s tax record. Everyone was searching for the tax record and it turns out that it was available as part of an ’anonymous’ dataset, made available by the IRS and again these were data that were released years and years ago.
They remained online and then suddenly they’re an extremely sensitive set of information that you can no longer meaningfully protect.
This goes back to what you were saying again about anticipating how certain techniques could be used in the future to potentially exploit these data.
YD: Actually, on this precise point, we know from cryptography that good cryptographic solutions are actually fully open and that the cryptographic solution is solid. I can describe to you the entire algorithm. I can give you the exact source code. The secrecy is protected by the process but the process itself is fully open.
If the security depends on the secrecy of your process, often you’re in trouble, right? And so a good solution actually doesn’t rely on you hiding something, something being secret, or you hoping that someone is not going to figure something out. And I think that this is another very important aspect.
And this perhaps goes back again, to the type of general misunderstandings which sometimes arise where someone might assume that because some data have to be kept private, as you were saying, that the documentation behind the process of ensuring that security also has to be kept private, when in fact you need open community standards that can be scrutinised and that people can build upon and improve. This is very relevant to our work in supporting things like data management plans, which require clear documentation.
We have reached our final question: There is arguably a tendency to focus on data horror stories to communicate the limitations of anonymisation (if applied for example without a proportionate risk-based approach for a research project). Are there positive messages we can promote when it comes to engaging with good or sensible practice more broadly?
YD: In addition to being transparent about developing and following best practices as we have just talked about, I think there needs to be more conversations around infrastructure. To me, it is not about someone coming up with and deploying a better algorithm.
We very much need to be part of an infrastructure building community that works together to instill good governance.
There are plenty of examples already in existence. We worked, for example, a lot on a project called Opal which is a great use case of how we can safely share very sensitive data for good. I think OpenSAFELY is another really good case study from Oxford and the CASD in in France as I already mentioned.
These case studies offer very pragmatic solutions, but are an order of magnitude better, both from the privacy and the utility side, than any existing legacy solutions that I know of.
[1] https://rea.ec.europa.eu/open-science_en
[2] https://www.washingtonpost.com/religion/2021/07/21/catholic-official-grindr-reaction/
Useful links:
CASD
https://www.casd.eu/en/le-centre-dacces-securise-aux-donnees-casd/gouvernance-et-missions/
CPG
Introduction to research data management
Opal project
OpenSAFELY
https://www.opensafely.org/about/
Open Science – European Commission
https://rea.ec.europa.eu/open-science_en