We were really glad to see James Howard (NHLI, Faculty of Medicine) announcing on Twitter that he’d published a Kaggle kernel to accompany his recent publication on MR image analysis for cardiac pacemaker identification using neural networks via PyTorch and torchvision. Sharing code in this way is a great way to promote open research, enable reproducibility and encourage re-use.
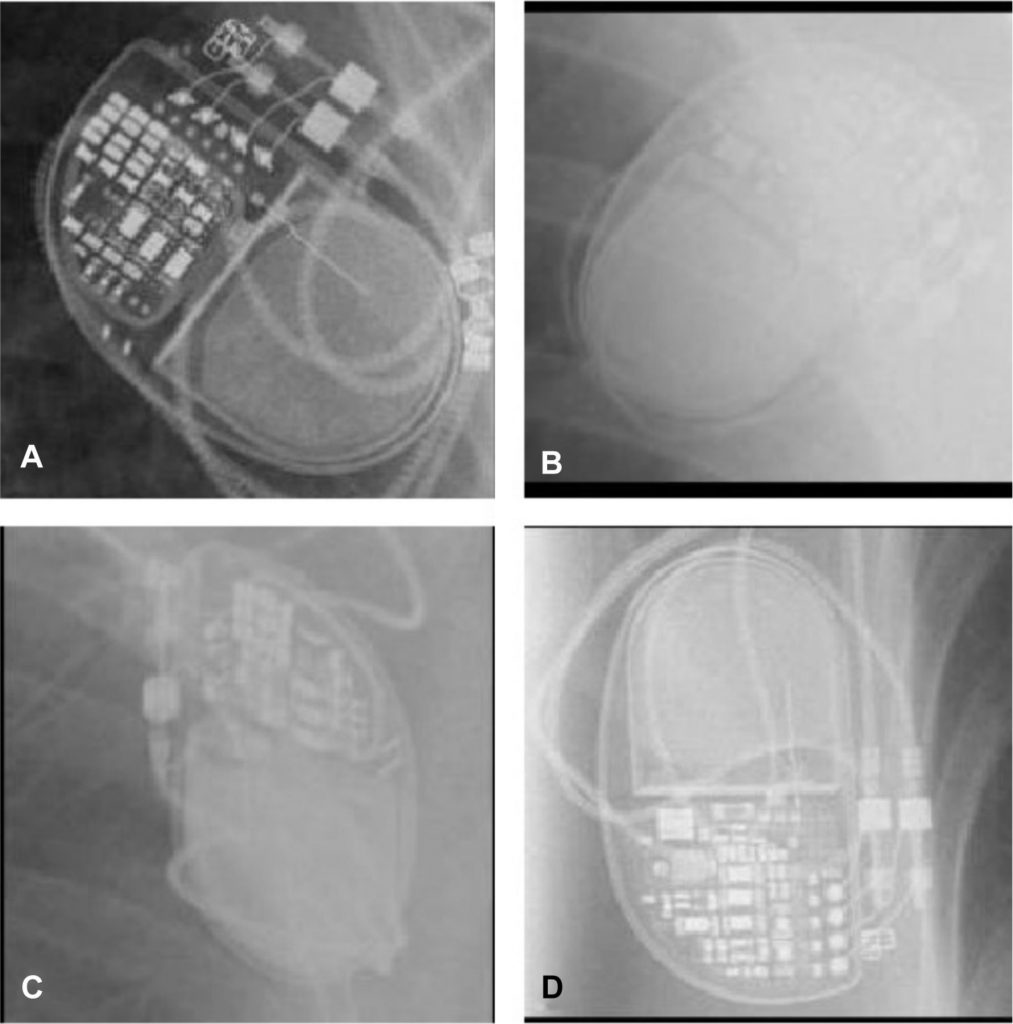
We thought it might be helpful to explain how to run similar notebooks on Imperial’s cluster compute service, given that it can provide some benefits while you’re developing code:
- Your code and data remain securely on-premise, thanks to the RCS Jupyter Service and Research Data Store
- You can run parallel interactive and non-interactive jobs that span several days, across multiple GPUs
With James’ permission we’ve lightly modified his notebook and published it in an exemplar repository alongside some instructions to run it on the compute cluster. We hope this can help others to use a combination of Conda, Jupyter and PBS in order to conduct GPU-accelerated machine learning on infrastructure managed by the College’s Research Computing Service – without incurring any cost at the point of use.
Many thanks to James Howard for sharing his notebook and reviewing our instructions